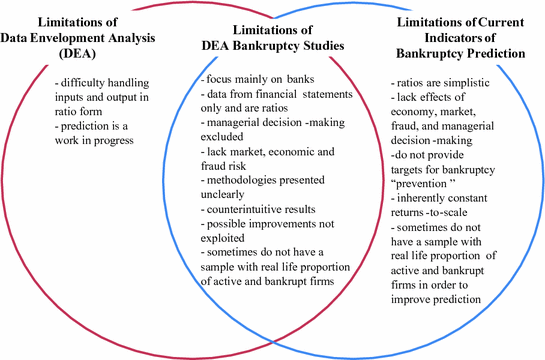
Introduction
Strategies for
forecasting payments to financial firms became an important issue in the 1960s
and have been extensively researched ever since. The increased emphasis on this
topic can be considered as a sign of progress and the strength of a given
national economy. High personal, financial and social costs forced by corporate
disappointment or non-payment have encouraged efforts to provide better
understanding and anticipation of termination. Given the extreme differences in
global spending, increased expectations for a corporate financial crisis will
provide useful information to leaders, for example, investors, lenders,
management executives, and the general public. Honestly, corporate financing
can be caused by a number of factors, for example, negative predictions,
unpredictable weather, incomes, and so on. Therefore, many current strategies
for recognizing corporate frustrations should be developed regularly. The
predictable types of Chapter 11 can be categorized into two general categories:
realistic and artificial canny (AI) methods. Beaver led the way with true
strategies, followed by Altman who used multi-discriminatory testing (MDA), and
developed stochastic models, for example, logit and probit. Still a reasonable
application of the truth
strategies are
constrained by their contextual assumptions, for example, equity, familiarity,
independence between indexing elements and previous useful frameworks that
point to legal variability and index flexibility. Over the past decade, various
investigations have used illegal methods in the prediction of chapter 11.
Currently, these strategies include (I) preferred trees including Interactive
Dichotomiser 3 (ID3), C5.0 and drug description and relapse (CART); (ii)
various mock organization (ANN) projects including multi-level understanding
(MLP), schematic mapping (SOM) and reading vector quantization (LVQ), (iii)
modification methods including asset calculation (GA) and more now the
development process - i -molecule swarm enhancement (PSO); and (iv) other clever
methods involving Support Vector Machines (SVMs). Among the clever strategies,
the selected trees form a fragment of 'AI' which is an important part of
man-made consultation [16]. Many selected drug statistics are used to address
collection issues. Selected trees can be used as a consolidation strategy to
incorporate the provided data guidelines and serve as an example of a forecast
of future stocks using RP) to see the closure of firms proposed by Marais et
al. Frydman et al. include the RPA in the prediction of the completion of the
comparison with the MDA. Cho et al. also analyzed selected trees and leased
thinking in anticipation of chapter 11. C5.0 is another selected tree counting
method developed in C4.5 by Quinlan(Yuliansyah, et al., 2017).
It incorporates
all the functions of C4.5 and uses new compounds for improved accuracy for
example to separate the evidence. In the ANN approach, MLP has extensive
applications in financial management. Since SOM and LVQ may not always be used
in the cash field, we are examining the expected completion of these two types.
In the development process, GA and PSO can enhance the capabilities and
opportunities for optima worldwide and redefine the limitations on outcomes.
Towards the end of the term, SVM has been applied to the use of a financial
forecast, for example, the FICO test, which is expected to schedule a time and
place for a security guarantee. Therefore, the purpose of this paper is to
compare these different collection methods and to identify the financial crisis(Vogel, 2019).
In this
experiment, integration models used direct discriminatory investigation (LDA),
regression strategy (LR), C5.0, CART, SOM, LVQ, SOM, GA, and -PSO. The main
objectives of this paper are to (1) improve the anticipation of systematic
misery, (2) increase the accuracy of these types of financial, non-monetary and
macroeconomic measures, (3) analyze general authenticity, intelligent, and
flexible calculations, and (4) ) have developed these mechanisms to create a
framework for pain predictability in order to provide data to financial
professionals and auditing organizations. Data used in our investigation was
collected from Taiwan Stock Exchange Corporation (TSEC) data sets. The remainder
of the paper is arranged as follows: Section 2 examines the writing of the same
survey of measurable, intelligent and flexible calculations approaching.
Section 3 provides a brief overview of the information organization and testing
model. Section 4 presents the results of the research and investigation. Place
5 presents our decisions.
Literature
Review
We will now take
a brief look at the transcripts of the actual and sensitive registration
process used so far to investigate problem situations and the forecast of
chapter 11. Specifically, we will be going into LDA, LR, C5.0, CART, SOM, LVQ,
SVM, GA - SVM, processes PSO - SVM and the short-term strategy of all the
methods in this section(Campbell, et al., 2011).
Statistical
Techniques
Non-discriminatory
inquiry (DA) is often used to order a number of ideas in the categories
described earlier. Cambell and Fung proposed a targeting strategy in the LDA to
distinguish powerful assumptions based on impact work. The LDA is a process
that recognizes that the information in each class is transmitted to the
Gaussian and that there is a novel framework for collaboration in each class.
The LDA similarly places the data in a modified space created by the
eigenvectors of the combined covariance lattice. So another example can be set
by editing it in a modified space and releasing the nearest centroid class, a
cycle known as direct selection limits. Apart from that, the LDA has a few
arrests. First, specific selection limits are not sufficient to handle the
Small Sample Size (S3) problem that occurs when the total number of preparation
tests is more modest than the vector size of the object. In the present case,
the internal phase of the grid distribution is particularly acute; it becomes
unthinkable for the LDA to address specific choice limits. Second, using a
single-class model may indicate failure and, a few models are eligible as a
rule. Third, we can have many consistent indicators. Given these issues, we
have replaced the discriminatory investigation with the LR, which is very much
in line with its allegations. LR is a retrospective way of predicting strong
ward dynamics. Unlike the LDA, LR does not require independent material to be
transferred or directly related, nor does it require equal distinctions within
each meeting. For LR models, the most reliable variable sits in a clear cutting
structure and has at least two levels. Free features can be in the form of
statistics or directly. Over time, many scientists have used LR to predict
financial closures. Laitinen and Laitinen used Taylor's model in anticipation
of completion and evaluated the use of the LR model to obtain data from a
Compustat data set. Premachandra et al. found that the LE envelopmented
information envelopment (DEA) test in anticipation of default. LDA and LR help
to measure different processes, and are reminded of our experiments(Vogel, 2019).
Intelligent
techniques
Tree Selection
(DT) is an indirect separation strategy that uses a lot of free features to
make the model into smaller modest groups. This process occurs on each branch
of the tree; selects independent variables with highly dependent relationships
and reliable variations as indicated by a particular model. Key figures for the
DT investigation model include C5.0 and CART. C4.5 calculation enhances ID3 in
relation to classification law and calculation process. Contrary to entropy
measures, it uses the rate-of-rate record as a measurement process in class
credits and as a result is less affected by the disadvantages of ID3 in those
subdivisions directly in the lower trees. The C5.0 calculation is a business
variance of C4.5 with improved regulatory years, and is shown as Clementine and
RuleQuest. The enhancement method also makes the C5.0 calculation faster and
generates more memory than C4.5. Motivation is a way to improve the results of
AI planning calculations. It sets the weight of each sample and, as the weight
rises, the effect of the sample is more noticeable on the selected tree. At
first, every example has the same weight. In all arrangements, another tree of
choice is produced.
The complexity
of each model is adjusted, with the aim of having the student focus on the
negatively divided tests by the selected drug developed at the beginning of the
final, which increases the complexity of these models and consequently gives
the C5.0 calculation an improved computational ability. The truck uses
tree-building figures that are clusters of events where predictive or criminal
situations occur. IN CART, a tree divider works by redistributing the scene
space into more soldiers. The trailer count shows a paired tree of choice,
unlike the ID3 that only makes two teens. The truck offers a number of options
that can be used with the data not included in the section to see which records
will have the given effect. Supported drug-based models, for example, CODE,
have a broader approach in which DT-based models do more on major issues and
can handle more modest data data than ANN models. Accordingly, our
investigation includes a combination of these two tree figures selected to make
reasonable recommendations in the forecast of chapter 11. Contrary to the above
figures, the collection process can be considered as taking into account the
expected issue of chapter(Kimmel, et al., 2018).
The SOM
calculation was originally introduced by Kohonen and is a neural collective
organization as in it a guide is developed to protect the geography of the
preparation information when the unit area transmits semantic data. Therefore,
the primary use of this is to collect data. Through SOM, a two-dimensional
presentation of the information space is often equated with a clear view. The
powerful use of SOM can be found in test information testing, project
acceptance, speech research, mechanical technology, modern diagnostics and
treatment, instruments and controls, and many different assignments. However,
little related testing centers around the prediction of financial distress. As a
result, this test will use the SOM strategy to anticipate noise as
cost-effective organizations. Another neural classification that is based on a
well-conducted study is a LVQ calculation marked by its heuristic understanding
and its rapid diversity in collecting activities. The LVQ neural design does
not include a layer of hidden units, so the neural organization consists of
only one layer of information and one layer of yield. In the LVQ calculation,
the weight vectors associated with each yield unit are known as codebook
vectors(Brown, et al., 2013).
The LVQ
calculation is a critical organization, and as a result, it is the production
units of each vector that are fighting among them to find a winner to a certain
extent. By the time the group is correct, the codebook vector of the nearby
harp has evolved into the preparation vector; if possible its base, is removed
away from the preparation vector. The LVQ calculation uses the Euclidean range
to find the winning unit, which adjusts its loads using the LVQ learning law.
Overall, LVQ is better than SOM in editing expectations due to targeted
reading. However, the use of the LVQ figure in cash flow planning has not been
adequately investigated and this study has expanded the SOM and LVQ processes
to create expectation models for both noisy and annoying organizations. The
problem of development may be difficult to meet with major research
limitations, but GA is often used to address the global volume goal, and can be
used to increase the capacity and improvement of many applications worldwide.
GA plays the development cycle in four stages: introduction, selection, hybrid,
and transformation. In the introductory section, the hunting area for all
possible programs is set to a limited number of strings. Each wire (called the
chromosome) has a comparative point in the interrogation space. The calculation
begins with the sub-settings selected from a number of settings in the tracking
space called individuals using harmoniously generated settings or using random
statistics. All sub-arrangements are evaluated using a well-known client work.
The function of fitness is to calculate to include the chromosome display. In
the optional category, a large number of people with high numbers of health
care are selected(Marson & Ferris, 2015).
This set
generates interest through different asset managers (e.g., hybrid or change).
In the hybrid phase, it operates in a trade-off of parts of the cable display
of several chromosomes (called '' guardians '') to create two chromosomes
(called '' children ''). In the transformation phase, it operates on a single
chromosome and one element is randomly drawn into a series of images, and the
local wire display is altered and so on. The downside of GA is that chromosomes
from a few well-balanced (but unqualified) people are likely to quickly
dominate society, causing them to coalesce into a much larger space. When
people come together, GA’s ability to continue to seek better arrangements is
exhausted enough. Another process to improve is the PSO calculation, first introduced
by Eberhart and Kennedy. The PSO incorporates the collection practices observed
in the running of feathered creatures, swarms of bees, and human behavior. As
evolving statistics, the PSO uses observations through a (so-called) community
of people (called particles) that are renewed from stress to cycle. Every
molecule has a respect related to well-being. These particles travel through a
space of advance.
speed requires
good preparation. The PSO calculation looks for the right motive by separating factual
data and social data between individual particles. The molecule refers to the
order of motion in a d-dimensional pursuit. Every molecule I’m talking about
and the position of the applicant, I remember the best value and the current
situation that brought that respect, called pbest. At a time when the molecule
embraces all people as its topological neighbors, the best is the best in the
world and is called the gbest. The PSO has two key managers: Velocity Renewal
and position renewal. Throughout the entire period, the whole molecule is
accelerated towards the gbest and to its own killing. Unlike GA, PSO is not
difficult to achieve, it does not have many parameters that require change, and
it meets quickly. In this way, we will incorporate these two growth computation
processes and the SVM model. Vector Support Machine (SVM) was recently
developed by Vapnik and his partners as a limited number of AI orders for
high-quality data(Somanath, 2011).
SVM uses a
straightforward model to create inconsistent class limits by setting
inconsistent input vectors in the space of high objects. SVM has also been
shown to be unaffected by an overdose, ultimately executing high assumptions in
solving various time and order problems. Preparing for the SVM is tantamount to
dealing with the problem of a compressed quadratic system so SVM planning is
always unique and around the world, unlike the preparation of various
organizations that require indirect performance that exposes the risk of intrusion
into neighboring minutes. The power of a different component can be selected to
get the best order results for a variety of configuration issues. Component
strengths can provide direct extensions from line to computational
inconsistencies and various fragments can be used in SVM models. These include
the vertical, polynomial, sigmoid, and proportional proportions (RBF)
components. The polynomial bit function is a static piece and is suitable for
issues where all the preparation details are placed. In addition, the RBF
component function has a few points of focus due to its limited and limited
response to all real information. In general, polynomial and RBF strengths are
applied to the SVM model(Mulford & Comiskey, 2011).
Since many of
the real issues are the problems of many laws, it would seem appropriate to use
a number of stone figures to seek redress. Accordingly, the paper implies good
management of end-to-end financial data sets including recorded Taiwanese
firms. This test includes a PSO calculation and a SVM split model. Proposed PSO
- SVM counts could reduce the chances of being caught in the vicinity and
improve the accuracy and ability to investigate globally. First, the PSO - SVM
figure introduces the particles and sets the parameters of the PSO including
object level, C, and γ. The PSO limit for computer health includes the number
of cycles, the speed limit, the number of particles, molecular measurements,
and weight. Second, the preparation cycle is also performed with an emphasis
counter initially set to 0. Third, the SVM model is operational from the setup
set, the test database is used to determine the accuracy and precision of the
model. If it is possible that the well-being of a molecule is superior to its
positive effect of the past (e.g. pbest), the previous positive effects of the
molecule are revived in the same way.
Besides, if
molecular well-being is better than global health (for example gbest), global
well-being is further enhanced. In the event that the final models are met,
then the cycle closes; however, the following emphasis is possible. Finally, by
the end of the preparation cycle, the PSO will acquire excellent SVM boundary
attributes, including base level, C, and γ. In addition, test results will also
receive test accuracy in test data with a modified SVM separator(Kimmel, et al., 2018).
Research
methodology and materials
Data
Our example
incorporates raw data from 200 TSEC recorded firms with a long probationary
period from January 2000 to December 2010. The fraction of the breakdown in
firms is almost 1: 3 to give better check results. For corporations, we have
compiled a balance over the next two years of debt default. All entities are
then divided into a preparation set and a test set, with a rotation ratio close
to 2: 1. All details are separated from the official budget reports which
include financial records, salary statements and payment methods taken from
TSEC's financial data sets, suggesting that the availability of these tests
could be summarized in companies outside Taiwan. Also, the proposed process and
test results could be useful in other global security exchanges(Dupont, 2019).
Variable
collection
The volatile
determination of the information vector is based on previous studies on
predicting financial distress by Kirkos et al., Spathis et al. , Fanning and
Cogger, People, Stice, Kinney and McDaniel, and Altman. There are a few
real-life strategies for selecting investigative materials including a free
t-test model, discriminatory testing, calculated recurrence, a selected drug,
and material investigations. However, many previous studies have found an
Altman Z-Score model that directs a limited amount of money to show in chapter 11
organizational opportunities and order equity as the five most important types:
productivity, monetization, action, influence, and termination. As a result,
the paper accepts material from previous studies found in the Taiwanese
Economic Journal (TEJ). We selected 50 items and added two rating classes:
non-financial estimates and macroeconomic estimates. Estimates were taken based
on their recurrence in writing and the behavior expected in the test; moreover
a few measurements were initiated in this study. We then used the PCA to issue
sensible items like climate donations. The tricks of all kinds of scale are as
follows:
Impairment rate:
long-term ability to deal with a fixed financial position, as recognized in the
cash flow statement, including current rate, basic analysis rate, amount of
income, amount of revenue, long-term liability income, added liability income,
and presentation revenue short-term and long-term bond ratio.
Results
Correlation
|
Interest Coverage
|
Financial Leverage
|
Debt/Equity
|
Financial Leverage
|
Debt/Equity
|
Interest Coverage
|
Financial Leverage
|
Debt/Equity
|
Interest Coverage
|
Financial Leverage
|
Debt/Equity
|
Interest Coverage
|
Financial Leverage
|
Debt/Equity
|
Interest Coverage
|
Financial Leverage
|
Debt/Equity
|
Financial Leverage
|
Debt/Equity
|
Interest Coverage
|
1
|
|
|
|
|
|
|
|
|
|
|
|
|
|
|
|
|
|
|
Financial Leverage
|
-0.36778
|
1
|
|
|
|
|
|
|
|
|
|
|
|
|
|
|
|
|
|
Debt/Equity
|
0.413518
|
0.470093
|
1
|
|
|
|
|
|
|
|
|
|
|
|
|
|
|
|
|
Financial Leverage
|
0.257519
|
0.478553
|
0.853249
|
1
|
|
|
|
|
|
|
|
|
|
|
|
|
|
|
|
Debt/Equity
|
-0.3104
|
-0.19955
|
-0.87792
|
-0.68294
|
1
|
|
|
|
|
|
|
|
|
|
|
|
|
|
|
Interest Coverage
|
0.181663
|
0.697507
|
0.845482
|
0.837801
|
-0.63331
|
1
|
|
|
|
|
|
|
|
|
|
|
|
|
|
Financial Leverage
|
-0.31054
|
-0.57498
|
-0.84207
|
-0.88285
|
0.612933
|
-0.75966
|
1
|
|
|
|
|
|
|
|
|
|
|
|
|
Debt/Equity
|
-0.35899
|
-0.54518
|
-0.78773
|
-0.8423
|
0.509588
|
-0.71595
|
0.982712
|
1
|
|
|
|
|
|
|
|
|
|
|
|
Interest Coverage
|
0.287204
|
0.566628
|
0.914546
|
0.919484
|
-0.73958
|
0.951225
|
-0.85305
|
-0.81214
|
1
|
|
|
|
|
|
|
|
|
|
|
Financial Leverage
|
0.427947
|
0.292719
|
0.868325
|
0.702781
|
-0.82277
|
0.569811
|
-0.77851
|
-0.75705
|
0.726876
|
1
|
|
|
|
|
|
|
|
|
|
Debt/Equity
|
0.401833
|
0.311814
|
0.853661
|
0.73094
|
-0.78794
|
0.586936
|
-0.77119
|
-0.75983
|
0.739092
|
0.991549
|
1
|
|
|
|
|
|
|
|
|
Interest Coverage
|
0.486765
|
-0.44098
|
0.426522
|
0.229093
|
-0.58431
|
-0.08519
|
-0.23015
|
-0.20714
|
0.149686
|
0.580926
|
0.534854
|
1
|
|
|
|
|
|
|
|
Financial Leverage
|
0.387722
|
0.00985
|
0.643772
|
0.558033
|
-0.77012
|
0.34001
|
-0.68402
|
-0.62909
|
0.539715
|
0.792532
|
0.741645
|
0.592343
|
1
|
|
|
|
|
|
|
Debt/Equity
|
0.39854
|
0.074583
|
0.695488
|
0.667129
|
-0.77222
|
0.448622
|
-0.7602
|
-0.71139
|
0.629566
|
0.783131
|
0.742505
|
0.534133
|
0.981872
|
1
|
|
|
|
|
|
Interest Coverage
|
0.784029
|
-0.29944
|
0.26359
|
0.215375
|
-0.10764
|
-0.06966
|
-0.41419
|
-0.46782
|
0.093752
|
0.366549
|
0.324954
|
0.553281
|
0.417898
|
0.413536
|
1
|
|
|
|
|
Financial Leverage
|
0.426078
|
0.157469
|
0.702157
|
0.343992
|
-0.74091
|
0.323228
|
-0.56768
|
-0.49614
|
0.424513
|
0.714726
|
0.621813
|
0.639405
|
0.723133
|
0.671083
|
0.481072
|
1
|
|
|
|
Debt/Equity
|
0.426631
|
0.16584
|
0.707945
|
0.348098
|
-0.74226
|
0.329283
|
-0.56981
|
-0.49939
|
0.426618
|
0.718991
|
0.62743
|
0.63774
|
0.716645
|
0.666357
|
0.479123
|
0.999668
|
1
|
|
|
Financial Leverage
|
0.807928
|
-0.35978
|
0.09135
|
-0.12507
|
-0.03236
|
-0.17888
|
-0.13165
|
-0.17967
|
-0.09742
|
0.261528
|
0.207222
|
0.324411
|
0.322244
|
0.258812
|
0.801302
|
0.452707
|
0.450405
|
1
|
|
Debt/Equity
|
0.219792
|
-0.29809
|
-0.49927
|
-0.54229
|
0.480054
|
-0.50503
|
0.346416
|
0.324263
|
-0.55439
|
-0.33571
|
-0.36327
|
-0.28423
|
-0.17449
|
-0.25738
|
0.295358
|
-0.09619
|
-0.10089
|
0.669778
|
1
|
Covariance
|
Interest Coverage
|
Financial Leverage
|
Debt/Equity
|
Financial Leverage
|
Debt/Equity
|
Interest Coverage
|
Financial Leverage
|
Debt/Equity
|
Interest Coverage
|
Financial Leverage
|
Debt/Equity
|
Interest Coverage
|
Financial Leverage
|
Debt/Equity
|
Interest Coverage
|
Financial Leverage
|
Debt/Equity
|
Financial Leverage
|
Debt/Equity
|
Interest Coverage
|
552.9078
|
|
|
|
|
|
|
|
|
|
|
|
|
|
|
|
|
|
|
Financial Leverage
|
-4.4712
|
0.267316
|
|
|
|
|
|
|
|
|
|
|
|
|
|
|
|
|
|
Debt/Equity
|
5.63323
|
0.14081
|
0.33564
|
|
|
|
|
|
|
|
|
|
|
|
|
|
|
|
|
Financial Leverage
|
7.835244
|
0.320154
|
0.63963
|
1.674296
|
|
|
|
|
|
|
|
|
|
|
|
|
|
|
|
Debt/Equity
|
-6.08958
|
-0.08608
|
-0.42436
|
-0.7373
|
0.696124
|
|
|
|
|
|
|
|
|
|
|
|
|
|
|
Interest Coverage
|
7.440958
|
0.628198
|
0.85325
|
1.888392
|
-0.92045
|
3.034384
|
|
|
|
|
|
|
|
|
|
|
|
|
|
Financial Leverage
|
-16.4057
|
-0.6679
|
-1.09605
|
-2.56655
|
1.148954
|
-2.97304
|
5.047684
|
|
|
|
|
|
|
|
|
|
|
|
|
Debt/Equity
|
-7.45542
|
-0.24895
|
-0.40307
|
-0.96262
|
0.375518
|
-1.10151
|
1.950028
|
0.780076
|
|
|
|
|
|
|
|
|
|
|
|
Interest Coverage
|
6.374038
|
0.276508
|
0.50008
|
1.122942
|
-0.58241
|
1.563924
|
-1.80892
|
-0.67701
|
0.890829
|
|
|
|
|
|
|
|
|
|
|
Financial Leverage
|
31.87952
|
0.479468
|
1.59373
|
2.880922
|
-2.1748
|
3.144574
|
-5.5412
|
-2.11829
|
2.173469
|
10.03671
|
|
|
|
|
|
|
|
|
|
Debt/Equity
|
12.28728
|
0.209648
|
0.64314
|
1.229932
|
-0.85491
|
1.329564
|
-2.25315
|
-0.8727
|
0.907149
|
4.085009
|
1.691089
|
|
|
|
|
|
|
|
|
Interest Coverage
|
58.32188
|
-1.16177
|
1.25911
|
1.510478
|
-2.48411
|
-0.75612
|
-2.63481
|
-0.9322
|
0.719886
|
9.377836
|
3.544086
|
25.964
|
|
|
|
|
|
|
|
Financial Leverage
|
5.832418
|
0.003258
|
0.2386
|
0.461932
|
-0.41106
|
0.378904
|
-0.98314
|
-0.35545
|
0.325884
|
1.606254
|
0.616994
|
1.930906
|
0.409264
|
|
|
|
|
|
|
Debt/Equity
|
3.8057
|
0.01566
|
0.16363
|
0.35056
|
-0.26165
|
0.31736
|
-0.6936
|
-0.25516
|
0.24131
|
1.00755
|
0.39212
|
1.10528
|
0.25509
|
0.16492
|
|
|
|
|
|
Interest Coverage
|
99.46059
|
-0.83525
|
0.82387
|
1.5035
|
-0.4845
|
-0.65468
|
-5.02036
|
-2.22913
|
0.477385
|
6.264995
|
2.279805
|
15.20982
|
1.44233
|
0.90603
|
29.10619
|
|
|
|
|
Financial Leverage
|
70.79795
|
0.575322
|
2.87459
|
3.145348
|
-4.36833
|
3.978766
|
-9.01263
|
-3.09653
|
2.831346
|
16.00074
|
5.714096
|
23.02326
|
3.269076
|
1.92583
|
18.34035
|
49.93552
|
|
|
|
Debt/Equity
|
39.5207
|
0.33779
|
1.61578
|
1.77445
|
-2.43976
|
2.2597
|
-5.04342
|
-1.73763
|
1.58629
|
8.97356
|
3.21436
|
12.80193
|
1.80614
|
1.06608
|
10.18322
|
27.82958
|
15.52
|
|
|
Financial Leverage
|
99.19371
|
-0.97126
|
0.27633
|
-0.84499
|
-0.14096
|
-1.62699
|
-1.54431
|
-0.82856
|
-0.48012
|
4.326131
|
1.407031
|
8.631094
|
1.076396
|
0.54879
|
22.57221
|
16.70347
|
9.26475
|
27.26277
|
|
Debt/Equity
|
5.295194
|
-0.15791
|
-0.29636
|
-0.71893
|
0.410372
|
-0.90136
|
0.797422
|
0.293434
|
-0.53612
|
-1.08971
|
-0.48402
|
-1.48386
|
-0.11437
|
-0.10709
|
1.63262
|
-0.69642
|
-0.40724
|
3.583108
|
1.049756
|
Conclusion
Following the
investigation of commonly referred to accuracy, accuracy, sensitivity, clarity,
and F measurement scales, we summarize four key bonds. First, following four
cycles of material investigations, 8 items with high standard values were
retained while more than 34 items were discarded. In any case, for less than
80%, our system is still ready to measure chapter 11 with high accuracy. In
addition, 8 non-monetary sizes and 1 combined macroeconomic file were deleted
by the PCA's primary investigation due to low data collection or collection of
non-cash forecasts. In line with these lines, our results from TSEC’s 200
recorded firms show that the allocation of money has a greater impact on the
performance of cash expectations than non-cash equivalents with macroeconomic
files. Second, as we approach the hour of real-time non-payment, the most
accurate expectations will be present in all planning statistics except the LDA
and LR statistics. Balanced cultural strategies are better suited to deal with
big data data without tolerating declining foreclosure. In addition, smart
strategies can help improve the performance of modest data sets and can be
influenced by large data sets.
Third, test
results with impairment tests indicate that C5.0 and CART have significantly
better expectations of degraded organizations. SVM and dynamic calculations can
increase the expected release of depleted organizations. Also, special research
shows that LDA, LR, C5.0 and CART have an unpredictable temporary forecast. the
performance of general organizations, and the actual statistics and DT give the
results of worse forecasts longer than the ANN and development statistics.
Finally, the paper suggests that SVM may be a more appropriate strategy than
conventional standard methods, DT and ANN in building a financial forecasting
model. GA and PSO strategies can also be integrated with SVM. Therefore, this
paper recommends that the PSO-SVM approach can be considered as a way to
predict financial distress. Additional testing is required for this team. While
the results of these assessments are obtained through planning calculations,
other sensitive registration strategies may also be applied to financial expectations.
In addition, our test results were obtained from TSEC community datasets.
Information from other security exchanges or sources of budget reports may be
attempted to verify and extend this approach. Finally, further research has
shown that different types of firms are affected by different financial levels,
and testing can lead to assessing the use of appropriate financial,
non-financial, and macroeconomic rates using the proposed method.
References
Brown, S., Bessant, J. R. & Lamming, R., 2013. Strategic
Operations Management. s.l.:Routledge.
Campbell, D., Edgar, D. & Stonehouse, G., 2011. Business
Strategy: An Introduction. s.l.:Macmillan International Higher Education.
Chandra, P., 2007. Financial Management. s.l.:Tata
McGraw-Hill Education.
Dupont, B., 2019. The cyber-resilience of financial
institutions: significance and applicability.. Journal of Cybersecurity.
Holmes, A., Illowsky, B. & Dean, S., 2018. Introductory
Business Statistics. illustrated ed. s.l.:Samurai Media Limited.
Jones, P. & Robinson, P., 2012. Operations Management.
s.l.:OUP Oxford.
Kimmel, P. D., Weygandt, J. J. & Kieso, D. E., 2018. Financial
Accounting: Tools for Business Decision Making. 9 ed. s.l.:John Wiley &
Sons.
Marson, J. & Ferris, K., 2015. Business Law. s.l.:Oxford
University Press.
Mulford, C. W. & Comiskey, E. E., 2011. The Financial
Numbers Game: Detecting Creative Accounting Practices. illustrated ed.
s.l.:John Wiley & Sons.
Needles, B. E. & Powers, M., 2010. Financial
Accounting. 11 ed. s.l.:Cengage Learning.
Rezaee, Z. & Riley, R., 2009. Financial Statement
Fraud: Prevention and Detection. 2 ed. s.l.:John Wiley & Sons.
Somanath, V. S., 2011. International Financial Management.
s.l.:I. K. International Pvt Ltd.
Vogel, F. E., 2019. Saudi Business Law in Practice: Laws
and Regulations as Applied in the Courts and Judicial Committees of Saudi
Arabia. s.l.:Bloomsbury Publishing.
Williams, J. R., Haka, S. F., Bettner, M. S. & Carcello,
J., 2017. Financial & Managerial Accounting. 18 ed. s.l.:McGraw-Hill
Higher Education.
Yuliansyah, Y., Gurd, B. & Mohamed, N., 2017. The
significant of business strategy in improving organizational performance. Humanomics,
33(1), pp. 56-74.